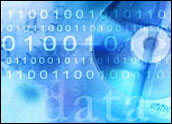
Today, the Consumer Data Integration (CDI) market has attracted several technology vendors from areas like ERP, CRM, data quality, and master data management. Unfortunately, while there is consensus that a CDI hub is critical for tying distributed customer data into unified views, there is rampant confusion on how best to implement such a hub.
Currently, there are four “styles” of CDI implementations. Before committing to a specific CDI style, organizations need to consider the fundamental business purpose of their current/future CDI hub, including: frequency of business change, scope/latency of unified views, legacy IT environment, operational versus analytical applications need, types of data sources and data governance policies.
Beyond these characteristics, an organization should choose a CDI style that is future-proof, i.e., it can adapt to merger & acquisitions, re-organizations and other systemic organizational changes. This involves four critical factors. First, the CDI hub architecture must adapt easily to changes over time, such as adding new business processes, data sources and applications. Second, it must allow for ongoing data stewardship/governance by both business and IT teams. Third, it must be an extensible IT platform in order to build new data views and services. Finally, it should be able to deliver these views in multiple modes — real-time and batch — to other systems at the performance and scaling requirements of the business.
The Four CDI Styles
Today, there are four different CDI styles which include:
- Custom-built data hub
- Fixed transaction hub
- Match and link cross-reference hub
- Adaptive transaction hub
Custom-Built Data Hub
This style reflects the historical way of building customer hubs using software tools and custom-coded rules. Building such a hub requires the use of an Extract-Transform-Load (ETL) tool to bring data into the hub’s data model from multiple sources and Data Quality (DQ) tools to cleanse and match similar records within the hub. These matched records are then merged based on simple, custom-coded rules, which generally results in the system choosing one record over another. Typically, ongoing code development is needed to integrate this custom hub to downstream systems, to make changes to match and merge rules and to add new data sources.
This tools-based approach is the least adaptable to business changes and has severely limited extensibility to new data sources.
Fixed Transaction Hub
Several application vendors like Siebel and Oracle offer this style of CDI hub which is built on a comprehensive but fixed data model and developed to support a specific set of applications. Despite its richness, this data model may not encapsulate all the relevant attributes required for unified customer views and needed by disparate downstream systems. To build the hub, all data from contributing sources is conformed to this data model, which may require extending the existing model and creating extensive ETL scripts to bring in data sources outside of the vendor’s model. This makes it difficult to upgrade the hub to the next version of the software product and may require specific tools to systematically update their normalized data model — which results in longer times for data loads.
Once data is cleansed, matched and merged, it becomes an operational data store (ODS) that serves up-to-date customer data, to multiple applications. The entire customer data — reference, relationship and transaction — required for a unified view is persisted in advance and tied to the hub’s fixed data model.
As a result, this style is best suited when there are only a few operational applications — identified in advance — supported by the hub’s fixed data model and when the business does not anticipate significant changes (i.e. additions of new applications/data sources).
Match and Link Cross Reference Hub
Also referred to as the “registry” style, this approach is offered by certain best-of-breed CDI vendors that historically provided matching tools. As a result, the data model of the customer hub contains only the selective attributes needed to match similar records across multiple data sources. The hub matches against these attributes and links the matched records to create a customer identity master store. This hub physically stores only the global ID, the cross-references (“links”) back to source systems and any mappings/transformations necessary to achieve semantic reconciliation.
With this style, standard DQ and ETL tools may be used to cleanse the source data and transfer the data from the sources to the system area for matching. Since there is neither any resolution of matched yet conflicting records, nor any history of past data states, this style cannot offer the best, resolved view of customer master data.
While this style offers beguilingly fast performance with low product “footprint,” it does so at the expense of critical functionality — primarily because little data is being stored or managed. Similarly, this style is highly scalable as long as functionality remains limited to persisting cross-reference links and no other data is stored/accessed (i.e. transaction or meta-data). Data stewardship is also limited since there are no merged master records to manage. Net, this style doesn’t create a full transactional hub for serving complete customer views, or an IT platform for developing and delivering data services to downstream systems.
Adaptive Transaction Hub
This style emerged most recently to address the limitations of the above approaches. With the adaptive style, the hub is built as a platform for consolidating data from disparate third party and internal sources and for serving unified customer views to operational applications, analytical systems or both. This hub is data-model-neutral and uses templates, tailored to each industry, which allows enterprise-specific data models to be implemented quickly. With the data model defined, data is loaded using standard ETL tools and cleansed via integrated third party DQ tools. Beyond just matching similar records, it merges matched records to build a “best-of-breed” master record that reflects the best-version-of-truth — at the cell-level — across multiple source systems. In effect, becoming the customer master or “system-of-record” for all systems.
This approach delivers a real-time hub that has a reliable, persistent foundation of master reference and relationship data, along with all the history and lineage of data changes needed for audit and compliance tracking. On top of this persistent master data foundation, the hub can dynamically aggregate transaction data — on demand — from different source systems to compose/deliver unified customer views to downstream systems. The scalability and performance characteristics of an adaptive transaction hub can also be altered — at multiple levels — to fit business requirements.
Once built, it delivers unified views to portals or embeds them within applications. Data can also be accessed through batch interfaces, published to a message bus or served through a real-time services layer. As a platform, new data sources can be readily added in this approach by extending the data model and by configuring the new source mappings, meaning that all legacy data hubs — CIF systems, identity and data cleansing hubs — can be leveraged to contribute their records/rules into the new transaction hub. Finally, through rich-user-interfaces for data stewardship, it allows exception handling by business analysts to keep it current with business rules/practices while maintaining the reliability of best-of-breed master records. Designed as a manageable, extensible and scaleable platform, the adaptive transaction hub serves as the most reliable foundation for delivering trusted unified customer views to all systems.
Before plunging into a CDI implementation, carefully assess your long-term requirements relative to the architectural approaches of each CDI style. Instead of getting enamored by a “big bang” solution or settling for one with limited capability, demand an evolutionary approach that does not jettison your investment in legacy data hubs, nor lead to a partial solution. The hallmark of a CDI hub is adaptability, manageability, extensibility and scalability — with a demonstrable path to achieving a fully transactional CDI hub built on service oriented architecture at the lowest total cost of ownership.
Anurag Wadehra is the Vice President of Marketing and Product Management at Siperian, a leading customer data integration and management provider.