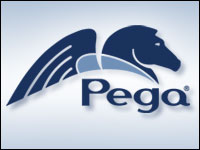
Pegasystems has rolled out Next-Best-Action Marketing 7.1. Built on Pega 7, it aims to provide marketing executives with the best customer service strategy — the next best action — for any given scenario, based on customer data captured by the system, along with rules configured by the user.
The differentiator between this and other platforms on the market, according to Ed Burek, director of product marketing at Pegasystems, is its self-learning functionality and ability to continually adapt to changing market conditions.

“We use real-time adaptive, predictive analytics that are constantly updating from the various inputs,” he told CRM Buyer.
“If a company is running multiple models it can take six to nine months for data scientists to update a model — but with self-learning, those models can be automatically adjusted,” Burek explained.
New Features
Pega Next-Best-Action Marketing 7.1 has enhanced outbound capabilities to provide better targeting of customers in the mobile channel. One new feature — Geolocation Targeted Marketing — defines specific geographic locations in order to offer appropriate real-time offers.
Another new feature, Outbound Offer Bundling, creates personalized bundled offers for customers.
There is also Apple Passbook integration in 7.1, allowing marketers to embed their offers into Apple’s virtual wallet.
Mobile In-App Marketing though Notifications integrates push marketing notifications to mobile applications.
Microsites & Landing Pages are part of the mix, providing tools to create microsites and landing pages.
Multiple Scenarios
With this new array of features, existing functionality, and the application’s flexibility in writing rules, Next-Best-Action can be configured for almost any use case, Burek said.
“The system itself updates and learns what should be the next best action regardless of the channel. A customer might start a conversation online and then switch to the phone, and the system will take that into account, but the analysis about behavior will be the overriding feature.”
For example, say a customer logs onto a banking website and is offered a home equity loan because the bank is aware of the customer’s mortgage and home equity. However, the customer doesn’t look at the online offer but instead pokes around the credit card offers for a while and then logs off without indicating any interest.
“The system might also know that this customer rechecks her bank balance at noon,” Burek said. “The system might then try to sell her again on the home equity line around that time by pushing out a notification to her social media page,” when presumably she is checking that too.
It might work — or the customer could get so irritated she blocks the bank from her social network. Either way, the system learns and accommodates that new insight.
Future Uses
This capability can be applied to any type of decision a company might want to make — not just marketing.
Some financial institutions are considering applying it to credit decisions, Burek said. For instance, someone with a lot of personal debt who applies for a loan might not have credit that meets the bank’s underwriting criteria. However, that customer might be married to someone who does have a high income and good credit. The person might then be offered a loan at a decent term with the spouse as a cosigner.
“The only limitations are the rules you put around it and what the predictive models look at and use,” Burek said.
It is much more common for Next-Best-Action to be applied in a marketing situation, although the potential for multiple scenarios exists.
For instance, a company like Expedia might decide it wants to use the system to keep inbound calls from swamping it at the IVR level, Burek suggested.
“The system would know that a certain customer always calls in two days before a trip to confirm the reservations,” he noted. “So the system could be configured to greet that person on the phone with a message, ‘Hello Mr. So-and-So. We see you are confirmed for your trip to Florida in two days. How else can we help you?'”